Deep tensor factorization and a pitfall for machine learning methods with Jacob Schreiber (#32)
April 29, 2019
In this episode, we hear from Jacob Schreiber about his algorithm, Avocado.
Avocado uses deep tensor factorization to break a three-dimensional tensor of epigenomic data into three orthogonal dimensions corresponding to cell types, assay types, and genomic loci. Avocado can extract a low-dimensional, information-rich latent representation from the wealth of experimental data from projects like the Roadmap Epigenomics Consortium and ENCODE. This representation allows you to impute genome-wide epigenomics experiments that have not yet been performed.
Jacob also talks about a pitfall he discovered when trying to predict gene expression from a mix of genomic and epigenomic data. As you increase the complexity of a machine learning model, its performance may be increasing for the wrong reason: instead of learning something biologically interesting, your model may simply be memorizing the average gene expression for that gene across your training cell types using the nucleotide sequence.
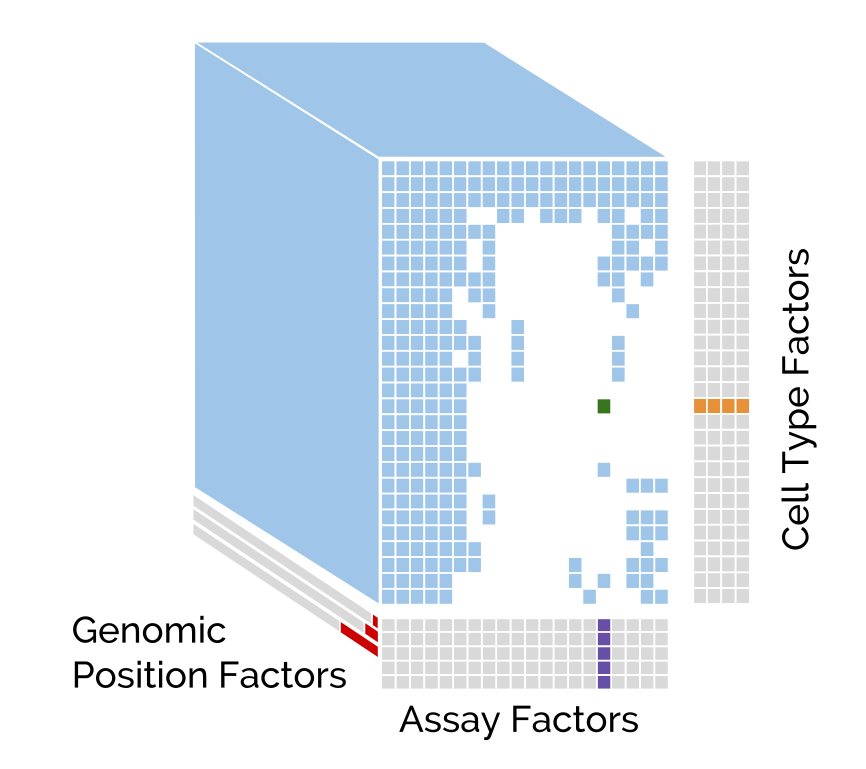
Links:
- Avocado on GitHub
- Multi-scale deep tensor factorization learns a latent representation of the human epigenome (Jacob Schreiber, Timothy Durham, Jeffrey Bilmes, William Stafford Noble)
- Completing the ENCODE3 compendium yields accurate imputations across a variety of assays and human biosamples (Jacob Schreiber, Jeffrey Bilmes, William Noble)
- A pitfall for machine learning methods aiming to predict across cell types (Jacob Schreiber, Ritambhara Singh, Jeffrey Bilmes, William Stafford Noble)
Music: Eric Skiff — Come and Find Me (modified, licensed under CC BY 4.0).
Subscribe to the bioinformatics chat on Apple Podcasts, Pocket Casts, Spotify, or any other podcasting app via the RSS feed link. You can also follow the podcast on Mastodon and Twitter and support it on Patreon.