The bioinformatics chat is a podcast about computational biology, bioinformatics, and next generation sequencing.
The bioinformatics chat is produced and hosted by Roman Cheplyaka. Several awesome machine learning-themed episodes have been hosted by Jacob Schreiber.
Subscribe to the bioinformatics chat on Apple Podcasts, Pocket Casts, Spotify, or any other podcasting app via the RSS feed link. You can also follow the podcast on Mastodon and Twitter and support it on Patreon.
Prioritizing drug target genes with Marie Sadler (#70)
December 21, 2023
In this episode, Marie Sadler talks about her recent Cell Genomics paper, Multi-layered genetic approaches to identify approved drug targets.
Previous studies have found that the drugs that target a gene linked to the disease are more likely to be approved. Yet there are many ways to define what it means for a gene to be linked to the disease. Perhaps the most straightforward approach is to rely on the genome-wide association studies (GWAS) data, but that data can also be integrated with quantitative trait loci (eQTL or pQTL) information to establish less obvious links between genetic variants (which often lie outside of genes) and genes. Finally, there’s exome sequencing, which, unlike GWAS, captures rare genetic variants. So in this paper, Marie and her colleagues set out to benchmark these different methods against one another.
Listen to the episode to find out how these methods work, which ones work better, and how network propagation can improve the prediction accuracy.
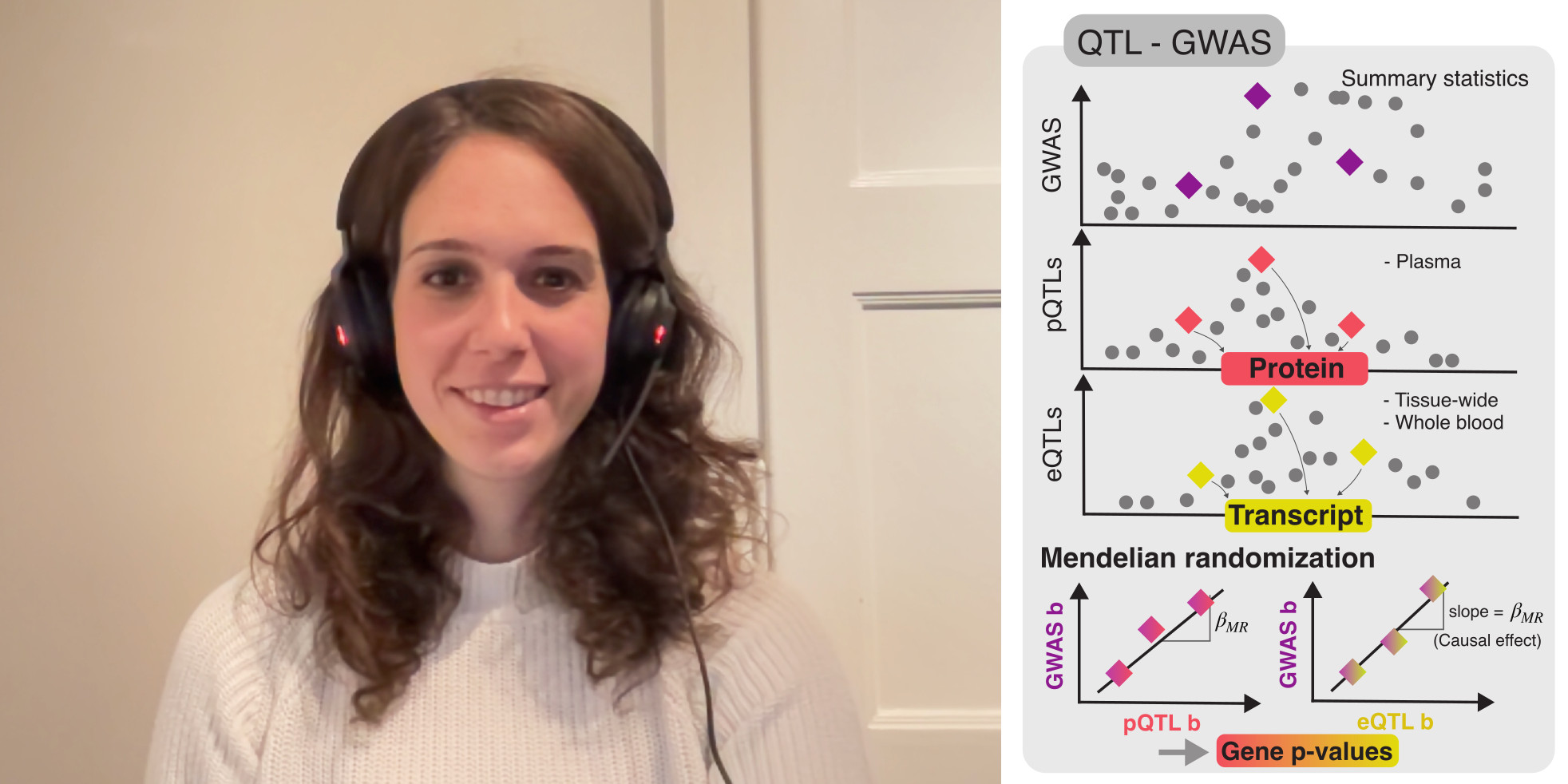
Suffix arrays in optimal compressed space and δ-SA with Tomasz Kociumaka and Dominik Kempa (#69)
September 29, 2023
Today on the podcast we have Tomasz Kociumaka and Dominik Kempa, the authors of the preprint Collapsing the Hierarchy of Compressed Data Structures: Suffix Arrays in Optimal Compressed Space.
The suffix array is one of the foundational data structures in bioinformatics, serving as an index that allows fast substring searches in a large text. However, in its raw form, the suffix array occupies the space proportional to (and several times larger than) the original text.
In their paper, Tomasz and Dominik construct a new index, δ-SA, which on the one hand can be used in the same way (answer the same queries) as the suffix array and the inverse suffix array, and on the other hand, occupies the space roughly proportional to the gzip’ed text (or, more precisely, to the measure δ that they define — hence the name).
Moreover, they mathematically prove that this index is optimal, in the sense that any index that supports these queries — or even much weaker queries, such as simply accessing the i-th character of the text — cannot be significantly smaller (as a function of δ) than δ-SA.
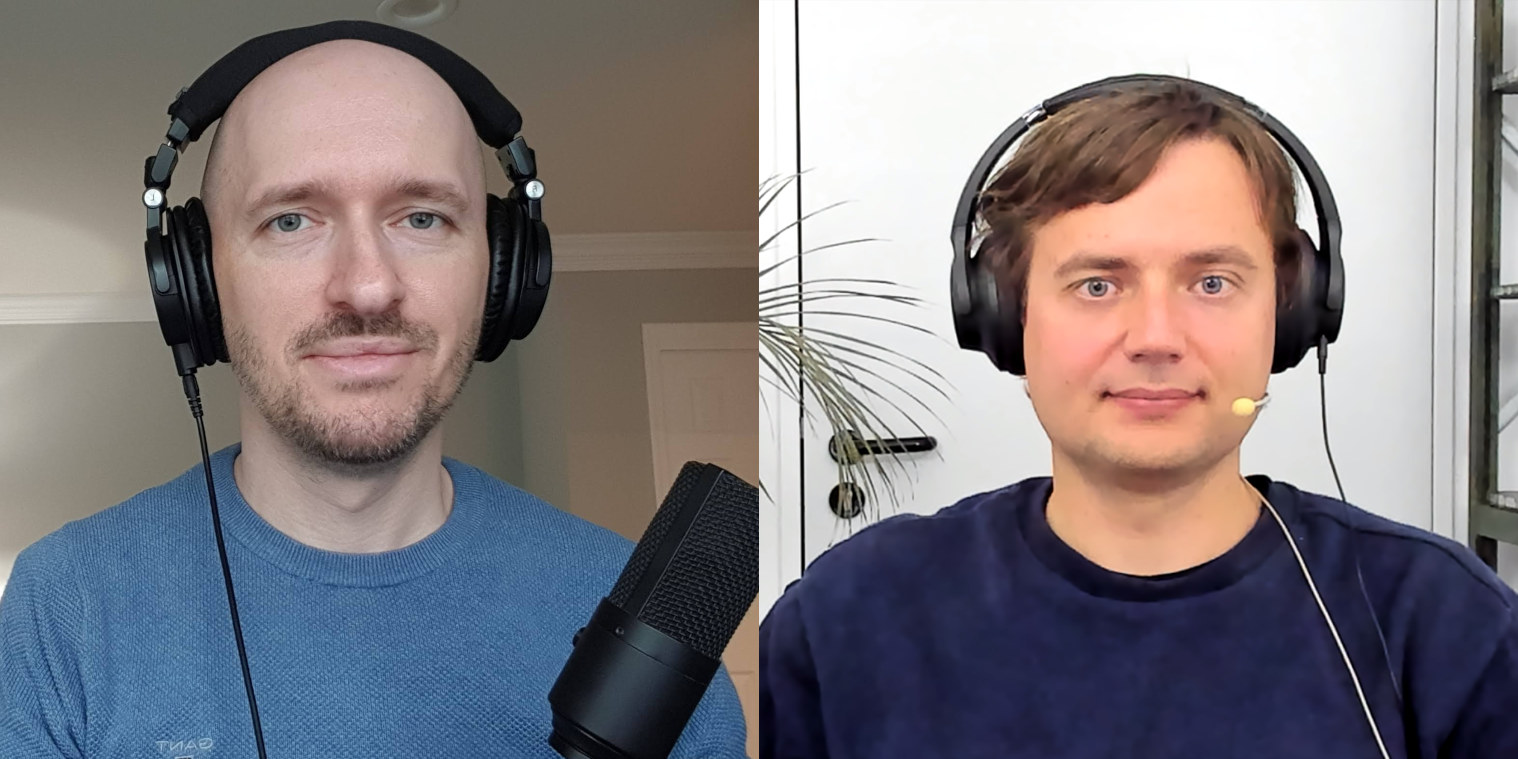
Phylogenetic inference from raw reads and Read2Tree with David Dylus (#68)
August 28, 2023
In this episode, David Dylus talks about Read2Tree, a tool that builds alignment matrices and phylogenetic trees from raw sequencing reads. By leveraging the database of orthologous genes called OMA, Read2Tree bypasses traditional, time-consuming steps such as genome assembly, annotation and all-versus-all sequence comparisons.
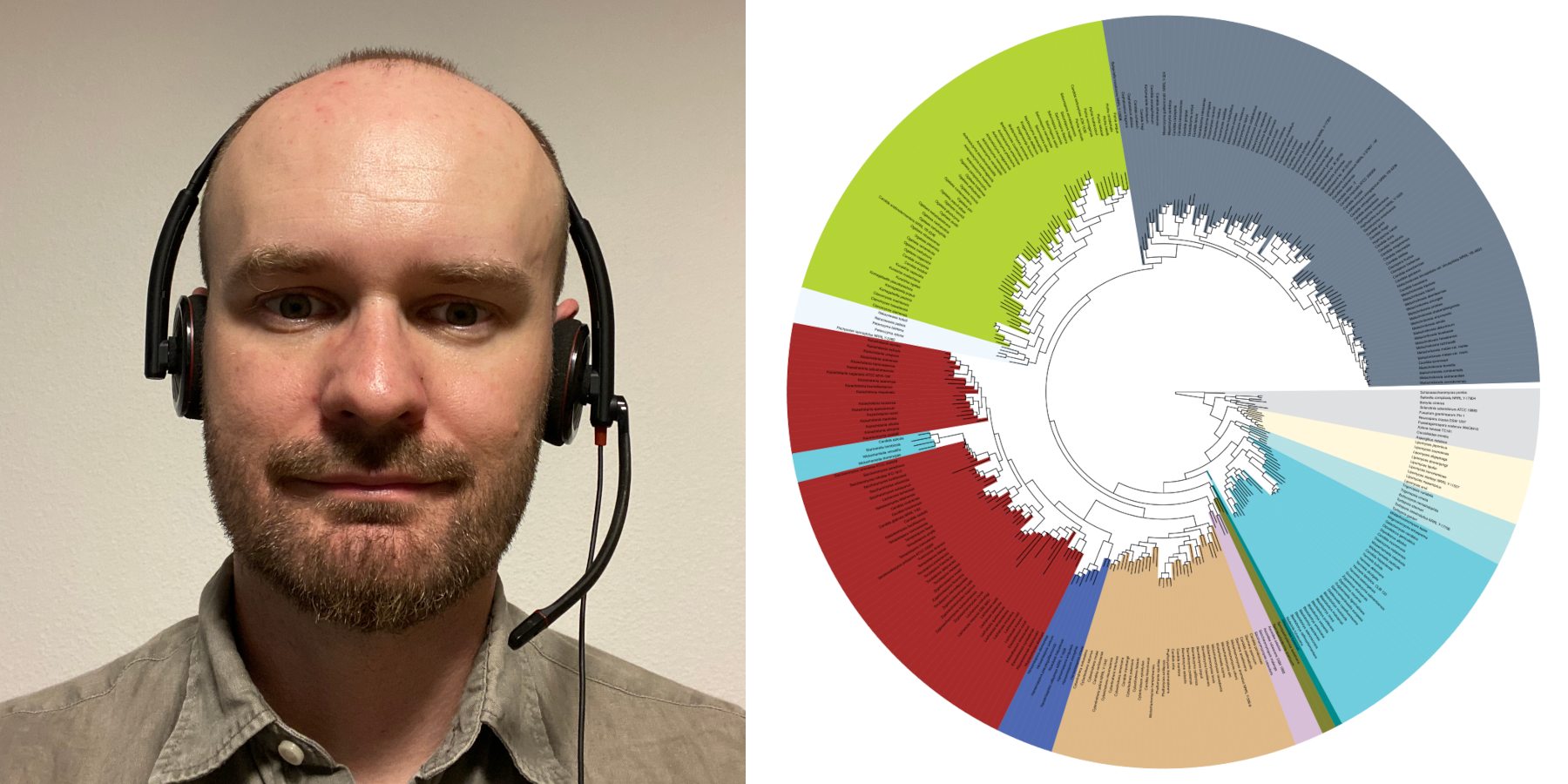
Previous episodes
AlphaFold and variant effect prediction with Amelie Stein (#67)
AlphaFold and shape-mers with Janani Durairaj (#66)
AlphaFold and protein interactions with Pedro Beltrao (#65)
Enformer: predicting gene expression from sequence with Žiga Avsec (#64)
Bioinformatics Contest 2021 with Maksym Kovalchuk and James Matthew Holt (#63)
Steady states of metabolic networks and Dingo with Apostolos Chalkis (#62)
3D genome organization and GRiNCH with Da-Inn Erika Lee (#61)
Differential gene expression and DESeq2 with Michael Love (#60)
Proteomics calibration with Lindsay Pino (#59)
B cell maturation and class switching with Hamish King (#58)
Enhancers with Molly Gasperini (#57)
Polygenic risk scores in admixed populations with Bárbara Bitarello (#56)
Phylogenetics and the likelihood gradient with Xiang Ji (#55)
Seeding methods for read alignment with Markus Schmidt (#54)
Real-time quantitative proteomics with Devin Schweppe (#53)
How 23andMe finds identical-by-descent segments with William Freyman (#52)
Basset and Basenji with David Kelley (#51)
Most Permissive Boolean Networks with Loïc Paulevé (#49)
Machine learning for drug development with Marinka Zitnik (#48)
Reproducible pipelines and NGLess with Luis Pedro Coelho (#47)
HiFi reads and HiCanu with Sergey Nurk and Sergey Koren (#46)
Genome assembly and Canu with Sergey Koren and Sergey Nurk (#45)
DNA tagging and Porcupine with Kathryn Doroschak (#44)
Generalized PCA for single-cell data with William Townes (#43)
Spectrum-preserving string sets and simplitigs with Amatur Rahman and Karel Břinda (#42)
Epidemic models with Kris Parag (#41)
Plasmid classification and binning with Sergio Arredondo-Alonso and Anita Schürch (#40)
Amplicon sequence variants and bias with Benjamin Callahan (#39)
Issues in legacy genomes with Luke Anderson-Trocmé (#38)
Causality and potential outcomes with Irineo Cabreros (#37)
scVI with Romain Lopez and Gabriel Misrachi (#36)
The role of the DNA shape in transcription factor binding with Hassan Samee (#35)
Power laws and T-cell receptors with Kristina Grigaityte (#34)
Genome assembly from long reads and Flye with Mikhail Kolmogorov (#33)
Deep tensor factorization and a pitfall for machine learning methods with Jacob Schreiber (#32)
Bioinformatics Contest 2019 with Alexey Sergushichev and Gennady Korotkevich (#31)
Bayesian inference of chromatin structure from Hi-C data with Simeon Carstens (#30)
Haplotype-aware genotyping from long reads with Trevor Pesout (#29)
Space-efficient variable-order Markov models with Fabio Cunial (#28)
Classification of CRISPR-induced mutations and CRISPRpic with HoJoon Lee and Seung Woo Cho (#27)
Feature selection, Relief and STIR with Trang Lê (#26)
Transposons and repeats with Kaushik Panda and Keith Slotkin (#25)
Read correction and Bcool with Antoine Limasset (#24)
RNA design, EteRNA and NEMO with Fernando Portela (#23)
smCounter2: somatic variant calling and UMIs with Chang Xu (#22)
Linear mixed models, GWAS, and lme4qtl with Andrey Ziyatdinov (#21)
Genome fingerprints with Gustavo Glusman (#19)
Bioinformatics Contest 2018 with Alexey Sergushichev and Ekaterina Vyahhi (#18)
Rarefaction, alpha diversity, and statistics with Amy Willis (#17)
Javier Quilez on what makes large sequencing projects successful (#16)
Optimal transport for single-cell expression data with Geoffrey Schiebinger (#15)
Generating functions for read mapping with Guillaume Filion (#14)
Bracken with Jennifer Lu (#13)
Modelling the immune system and C-ImmSim with Filippo Castiglione (#12)
Collective cell migration with Linus Schumacher (#11)
Spatially variable genes and SpatialDE with Valentine Svensson (#10)
Michael Tessler and Christopher Mason on 16S amplicon vs shotgun sequencing (#9)
Perfect k-mer hashing in Sailfish (#8)
Allele-specific expression (#6)
Relative data analysis and propr with Thom Quinn (#5)
ChIP-seq and GenoGAM with Georg Stricker and Julien Gagneur (#4)
miRNA target site prediction and seedVicious with Antonio Marco (#3)
Single-cell RNA sequencing with Aleksandra Kolodziejczyk (#2)