Generalized PCA for single-cell data with William Townes (#43)
March 27, 2020
Will Townes proposes a new, simpler way to analyze scRNA-seq data with unique molecular identifiers (UMIs). Observing that such data is not zero-inflated, Will has designed a PCA-like procedure inspired by generalized linear models (GLMs) that, unlike the standard PCA, takes into account statistical properties of the data and avoids spurious correlations (such as one or more of the top principal components being correlated with the number of non-zero gene counts).
Also check out Will’s paper for a feature selection algorithm based on deviance, which we didn’t get a chance to discuss on the podcast.
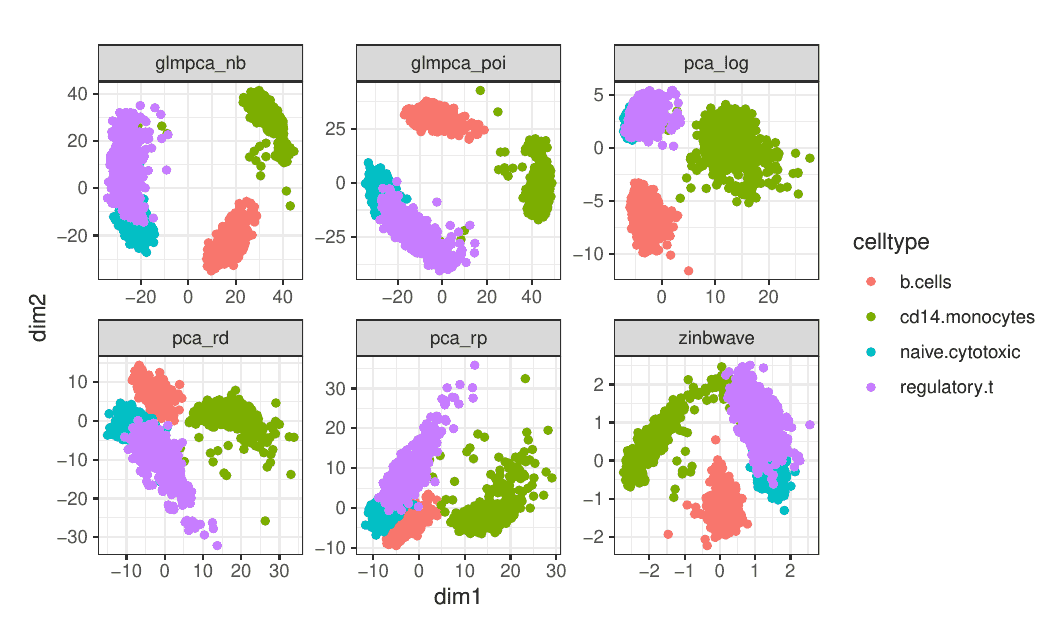
Links:
- Feature selection and dimension reduction for single-cell RNA-Seq based on a multinomial model (F. William Townes, Stephanie C. Hicks, Martin J. Aryee, Rafael A. Irizarry)
- GLM-PCA for R
- GLM-PCA for Python
- scry: an R package for feature selection by deviance (alternative to highly variable genes)
- Droplet scRNA-seq is not zero-inflated (Valentine Svensson)
Thanks to Daniel J. Kearns from Princeton University’s Instructional Support Services for his help in recording this episode.
Music: Eric Skiff — Come and Find Me (modified, licensed under CC BY 4.0).
Subscribe to the bioinformatics chat on Apple Podcasts, Pocket Casts, Spotify, or any other podcasting app via the RSS feed link. You can also follow the podcast on Mastodon and Twitter and support it on Patreon.